Can Artificial Intelligence Solve Manufacturing Problems?
Why Andrew Scheuermann thinks AI will be the assistant every engineer has needed.
by Mike Buetow
We in electronics design and manufacturing know automation is part and parcel of what we do, but while the landscape has changed, be it the transition from mechanical drawings to CAD tools with their autorouters or from manual and semiautomatic printers and placement machines to lights-out factories where cobots have replaced operators, the industry still has a long, long way to go.
To help with perspective on this emerging technology, we interviewed Andrew Scheuermann in February. Scheuermann, along with his business partner, Tim Burke, is cofounder and CEO of Arch Systems, a Silicon Valley-based developer of software tools that collect raw machine data and use predictive and analytics to calculate manufacturing key performance indicators or KPIs.
Scheuermann has published numerous scientific papers in the areas of semiconductors, electronics manufacturing, and renewable energy. He has a Ph.D. in materials science from Stanford and is also part of StartX, a startup accelerator for company founders who are affiliated with Stanford and that has invested over $200 million in various crump companies including 13 that are now valued at over $1 billion.
The interview, which took place on the PCB Chat podcast, is edited here for length and clarity.
Mike Buetow: In practical terms, how do you respond when someone asks what AI means when it comes to manufacturing?
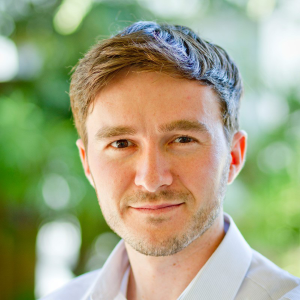
Andrew Scheuermann: Ah, the ultimate question. It’s so hard to say what it is, because there is not a clear definition. AI in manufacturing is doing something in the factories that only humans could do before. The reality is that it’s kind of this moving line, and manufacturing has always been this area where people have been pro-automation, pro-improvement, Lean manufacturing. Of course we’re continually improving. We’re not going to solve the problems of yesterday; we’re going to solve the problems of tomorrow.
I think it’s hard to say “what’s AI in manufacturing?” because it’s actually a moving target. Being a little more specific, I think AI is using data and algorithms to guide people to improve manufacturing, and again, do it in a way that humans alone could not do it before.
MB: We find new technology innovations solving problems of today, but also sometimes presenting problems that were unforeseen and introducing ones that didn’t exist. I want to keep that in the back of our minds as we proceed. But first, how would you describe the current status of implementation?
AS: I like to break things up in use cases in manufacturing and think about where is AI as it relates to vision systems, where is AI as it relates to KPIs like predictive maintenance, where is AI as it relates to quality.
For the last question we were talking about how it’s a moving line. Let’s take AI as it relates to vision and inspection. That’s a big area in manufacturing. There was a time when it’s, “Oh, this computer can identify this is a red apple or this is a green apple,” and it’s like, “Oh my gosh! That’s AI.” The conversation today is when people try to figure out what’s the state of implementation in computer vision. Is it rules-based, or is it AI-based? And what they mean by AI is not the rules that I currently understand, like “red and green” or “I detect which color it is and I just say it.” That’s super easy. Even: Is the chip here or is the chip not here? Well, that can be rules-based. “I know it’s supposed to be exactly in this spot, and I don’t see a chip; that’s a rules base, that’s not AI.” But AI is really generating a new set of more complex rules.
For example, in computer vision, the state of implementation that I hear everybody talking about now is a use case like the prior rules-based computer vision. It was able to do a decent job of detecting issues, for example, on the PCBA line, and it would send the ones that it wasn’t sure about to a review room where there’s a human who’s looking at the picture and going, “Yup, chip is missing. Oh no, actually chip is there. No fault found.” And now it’s, “OK, what if we don’t need a human to do that step because the algorithm is more accurate?” It can detect even without being told exactly where a chip is. It can do unsupervised things. That’s an example of where the state of implementation is. We know how to say if something’s red or green. We know how to say if a chip is here or not here if we’re expecting the position. But now we’re starting to be able to take images of boards and things that change more often. We don’t necessarily have the design data and kind of just detect anomalies like it’s different from the last image that went through.
What about maintenance? Predictive maintenance is getting really exciting in a number of areas. Now that we can pull process data out of the machine. We can get all the error codes. We can get pressures, temperatures, current signals. We can take that data and stream it in a way that we were already doing an SPC tools for quality. We were already looking at a signal and saying, “The resistance is too high or too low on this board. You need to rework it.” But now we can look at that same kind of flowing data from, say, the pick-and-place so the solder printer and the selective solder and other things and we can predict a maintenance problem by the parameter getting out of control. Again, that’s kind of the state of the implementation using error codes and parameters and predicting that things are out of bounds. We like to think about things on a per use case basis and what is that leading edge of using data and algorithms to predict things and guide better actions in the factory – at Arch we call them “intelligent actions” – that in the past could only be done by humans.
MB: What you’ve described sounds to me like a very linear, almost orderly process of implementation. I think one of the big questions on many engineers’ minds is the issue of what I would call “impact versus replace.” I want to stay away from the ethical questions of AI because that could be an interview in its own right and in any case, it’s not something that those of us in the trenches of our industry will have much influence over. But my question is, should we view AI as “just another form of automation?”
AS: I would not. I look at it as something new. It is correct to say it is a form of automation. But I think “just” another form of automation is simplifying, because it often automates things that weren’t necessarily being done that way before.
That’s something we talk about at Arch. It’s like, “Oh, right now the human picks up a part and it puts it here. Oh, now a robot can do that.” Okay, that’s automating a job that was being done before, but was I doing predictive maintenance before? Not necessarily; I didn’t know when it was going to fail so I wasn’t even doing that like could I have done that. Well, what if a human went and manually downloaded all the data or they could have every sensor machine has a log file or something somewhere? What if they had manually grabbed all of that, thrown it into a spreadsheet, crunched some math, and then by the time they did it, it’s like, “Oh, the machine’s already failed. I ran out of time to do my math.” There are these complex problems that weren’t actually being done before. We’re automating what in your mind a person could have done but were they actually doing it? Yeah, maybe they didn’t possibly have time to predict that.
Back to the computer vision example, that is a case where potentially there is a human being replaced and so people are calling that use case “automation,” and that makes a little more sense because there’s a person in the control room and [now] maybe there won’t be a person in the control room. There used to be a person at the cashier register to check out your goods and there’s not anymore. That makes sense to call it automation. But things like predictive maintenance, predictive quality … a lot of things that go in predictive analytics are adding new capabilities that we didn’t have time to do as a human, even if it was theoretically possible.
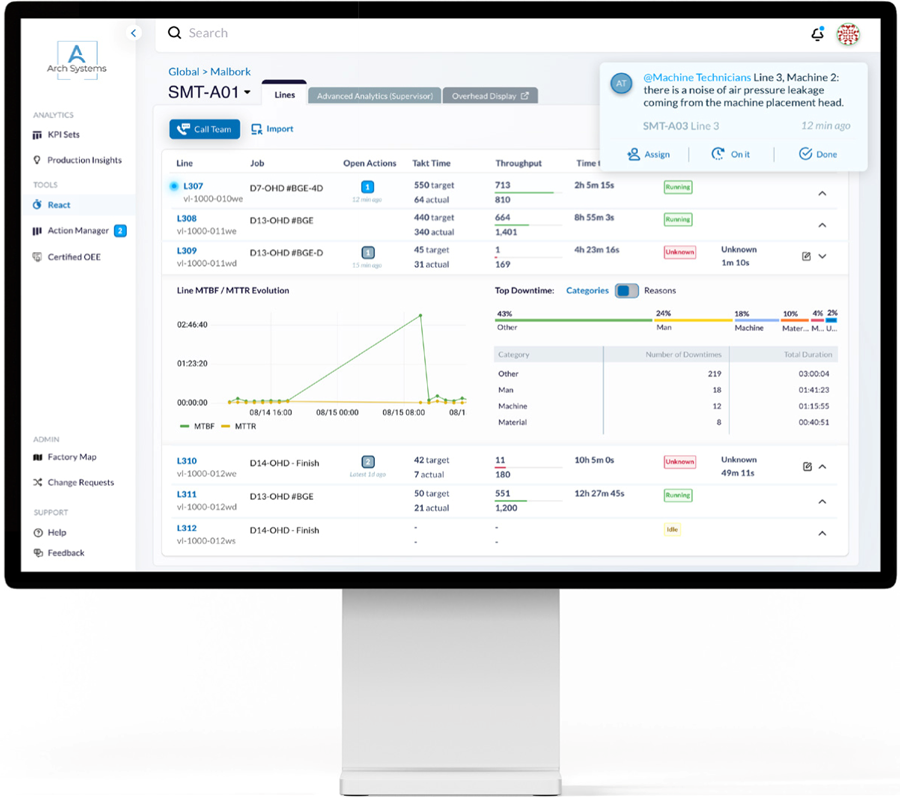
MB: Neil Thompson, who is the director of the future tech research project at MIT’s Computer Science and Artificial Intelligence Laboratory, has argued that it’s not enough for AI systems to be good at tasks now performed by people, but the system must be good enough to justify the cost of installing it and redesigning the way the job is done. He says, “there are a lot of places where humans are a more cost-efficient way” to do that. Would you agree?
AS: Absolutely, yeah, I strongly agree. And I think that’s kind of like a low bar for AI. Let’s look for the little problem a human is doing and replace it, like the example I gave with predictive maintenance. Some of the stuff we like to do at Arch, it’s not solving a problem that doesn’t exist, but it’s solving a problem that does exist that people just don’t have any idea how to solve, such as people aren’t doing it because they can’t possibly run to all these machines, grab the data, put it together, and have predicted this thing before the defect was already there or before the machine went down. That’s extremely exciting.
Are there things that humans are still better at than machines? Absolutely. I’m a complete believer in that. I think that’s one of the exciting things about some of the newest AI, like the large language models, ChatGPT, that everyone’s been using. It really is good as a copilot and it’s being built into tools like ours and others where it’s directly pairing with people.
It’s not like, hey, we’re not even going to talk anymore. We don’t have the AI talk for us. It’s, no, we’re going to talk to the AI to better understand the data. Because Arch was already pulling data from all these machines you didn’t have time for into a predictive maintenance. But then you still had to go through our interface and find it or you had to know the right dashboard or you had to get training on that dashboard. What if now you can just talk to it and it takes you right to the dashboard or explains it for you? Everybody can have an assistant now. That’s one of the really exciting parts of this most recent wave of AI and it has everything to do with amplifying people.
MB: For an engineer, a designer, an operator, what skills are needed now and for the next generation to fully realize the potential of AI?
AS: Back to that last thing I said, that AI is kind of becoming an assistant for everyone. In the past this was something that maybe only really rich people have. If you’re just super rich, someone’s doing your calendar and helping with your kids and helping run your business and getting your food, whatever it is. Now more and more with AI, each of us can have an assistant, so a factory engineer can have an assistant, a factory operator can have an assistant. The GM already had assistants, but they have even more assistants. So that’s the first thing I would say when you think about what skills do you need.
How do you use an assistant? Well, it’s actually a hard thing to do if you’ve never practiced it. I don’t know how to delegate. I don’t know how to share my work with someone who’s healthy. I don’t know how to ask the right question. So that “ask the right question” is called prompting with the new language models, for example, and before language models it was, I need to know how to click on the right dashboard and even get the right data, so both knowing how to navigate through web browsers, how to Google search, how to do a basic Boolean argument and now how to prompt, which in in a way is even easier because you prompt an LLM [large language model] just like you talk, but there still is a bit of an art to it. There’s a good way to prompt and a bad way to prompt. So those are the kinds of skills that are becoming even more important.
MB: It sounds like one of the skills that people will need may be precise communication skills, at least to start.
AS: Exactly. Precise communication skills. Imagine an operations manager and engineer and factory and version 1 of the person can’t articulate the problem at all. The only technology they could use is a big red button that says, “Help me.” And now the AI on the other side has to be as smart as possible. They click the button and I have no idea what’s going on here. I can’t see anything, can give no notes. No description. No pictures, just Help Me, and technology vendors like us, we want to build something that powerful so you could literally just do that. But if you think about the skilled side, what about the person who could precisely describe what’s happening? So yeah, there’s a bunch of data going on and they know how to grab this dashboard, that dashboard, because again, these AIs are always improving. They look at two pieces of information and they’re like, “I think I have a problem at the printer and I saw this before here. Can you help me? What did we do last week when we had a problem with the printer?” And all of a sudden they’re going to get way more out of that technology than the person that’s just “Help me” and hits a button. So exactly, being able to describe your problem, know how to use your tools, and then kind of meet AI right at the interface.
MB: It sounds to me like AI really kind of replaces your Google search or what have you, because it’s so much more comprehensive. Google can give you an answer to a question. AI can develop a whole strategy. Is that a fair way to put it?
AS: Yeah, I think that was said well.
MB: Let’s look to the near future. Joseph Fuller is a professor at Harvard Business School where he studies the future of work. Fuller said that the latest AI innovations will have dramatic impacts in some areas related to our industry but not so much in others. Let me give you an example. He thinks generative AI is already so good at creating computer software that it will reduce the demand for human programmers. It’s relatively easy to teach an AI how to write software; just feed it a lot of examples created by humans. But teaching an AI how to manufacture a complex object is far more difficult. What Fuller says is, “I can’t unleash it on some database on the accuracy of manufacturing processes. There is no such data, so I have nothing to train it on.” Given that, where do you think AI will be in electronics manufacturing three to five years from now?
AS: He says AI is extremely good at programming. I agree. It’s amazingly good. I love programming with AI. I’ll never program without AI myself. It upped my level overnight when I could talk to it. Back in school I studied material science, but I did some computer science too, and in the courses at Stanford, we would do peer programming because when you program with a peer you do everything better, so that kind of copilot like it is a perfect marriage with coding. So that part’s exactly right.
His second comment – this isn’t going to work in the factory because there’s no data – well, I’m going to agree and disagree. I’ll do the disagree first. There’s a lot of data. Arch, we’re still in the grand scheme of things a really small company. We process more than a billion data points today from 150 to 200-some factories of 10,000 simultaneously connected machines and systems that feed our systems all the time. There’s a tremendous amount of data in manufacturing now. Where I agree with him is the nature of the data and manufacturing, it has to be split into these pieces because this is the data specific to how I make this, for instance, iPhone circuit board, and this is for this engine control unit, and this is for a missile, etc. All the different products that we’re building are reasonably different and I think more different than just like another piece of code.
A lot of people have talked about how these language AIs can go all the way through high school and even a little bit into college and they can pass the SATs and they can pass all these standardized tests extremely well. They’re good at general knowledge because there’s so much of that digitized out there on the internet, you feed it in and they can regurgitate it amazingly well and even build new creative combinations of existing knowledge. But if you go to manufacturing: Okay, how do I build this product that I have right now? How many copies of the data for that exact product exist out there? Nothing at all compared to the data to solve a vocabulary quiz, and so again, this is where I’m going to agree and disagree.
So I disagree that there is no data. There’s a massive amount of data. But is there a ton of repeat data on exactly the same products and problems? No. What we have to do in manufacturing is combine these generic AIs that are being built with the specific data of each manufacturer’s problem, which needs to be kept private and secure only to them, so they can combine these things in a controlled environment and then they can get the best use of AI combining general AI and their specific data for their problems.
MB: In SMT we look at outputs and variations of the product being built but this is really where Arch comes in. You can mine all sorts of data that in some cases manufacturers probably don’t even know they have. For instance, I watched one of your YouTube clips and you talk about the motor voltage and movements of a given machine, even if it’s a so-called dumb machine. It begs the question though, how are companies going to manage the data ocean without drowning and what specifically are you doing to help?
AS: [The YouTube video referred to] a sewing machine at Nike. We were able to take the current data out of it and turn it into a stitch model, which kind of makes sense but people were totally shocked: “Wait, this isn’t a smart machine but you can say exactly where the stitches are.”
If you were going to build a smart sewing machine, you’d have to do the same thing. You’d have to use the data, the current, the pedals, the everything, and create a model of how the stitches are going, and then once you had that stitch model we were able to predict a good pattern from a bad pattern by comparing to the expert sewers that do extremely well. There’s a sewing machine called a Strobel that kind of sews to the side, so you can do the heel of a shoe. That’s one of the hardest parts. It takes nine to 12 months to train someone to do it well. You can now immediately compare an expert sewer to a non-expert sewer and help them see when they were doing a non-ideal pattern. That was just kind of one really cool example.
In SMT there’s a lot of similar things. There’s this tremendous amount of rich data in the pick-and-place machines. You know every kind of mispick that’s happening on an exact track related to an exact material related to this head related to this camera. Same things on the printers and the AOIs and the ovens, and we’ve been able to correlate data across machines that people didn’t necessarily expect in really cool ways.
There’s parts of what we do at Arch that are just genuinely hard and the customer asks and we go, “Yeah, that’s hard and even for us it takes time,” but there’s other parts where something’s actually extremely easy and powerful that people thought was hard and this is one of them. You asked about the data ocean. Every single one of our customers is floored by how effectively we can grab all this manufacturing data and put it into a lake or an ocean at extremely low cost and sustainability. At the end of the day, you know all these files, all these data parameters and everything can be harnessed, they can be compressed way more than people think and it completely makes sense these days to put it into an ocean and then be able to use these advanced analytics to Google search through it; prompt AI to talk through it and correlate it with old school fantastic statistics as well and discover all the things inside that data when you finally unify it.
MB: You mentioned sustainability. Can you define what it means in this context?
AS: In that context I was talking about cost and business sustainability more than energy or carbon sustainability. But of course there’s a link, like if you’re spending a tremendous amount of data on server farms. They’re extremely hungry from a CO2 perspective, but business sustainability also means are you doing something that you’re going to keep doing. So many times in the past companies invested in technology. It wasn’t sustainable. They couldn’t maintain it. Then they had to dump it and that investment went to zero, and this is one of the areas in decades past people built a data lake or a data ocean. It cost a tremendous amount of money; they didn’t somehow magically create AI. AI didn’t just emerge from the data. It wasn’t that you just put it in the data lake and clicked your fingers and AI came out of it, so a lot of people lost money in the past doing this but that’s where you have to understand the difference between past lessons and the future, because technology is changing so fast. Today, it is possible to build a data lake, a data ocean at extremely low cost that is sustainable to manage. It is still true that AI doesn’t just magically come out of the data once you put it in the same place, but it is extremely low cost to put it together that then allows you to do AI with it, and that AI is working very well.
MB: Who owns the data that you’re mining? Obviously the manufacturer, your customer, is developing products and you’re able to take all those zeros and ones together and make sense of it all, but in terms of what you do with that in the aggregate afterwards, improving your tool or improving the universe of your customers, so that things that have been learned are in the aggregate used to help, perhaps even their competitors. Where does that go and how should we think about that?
AS: Customers own their own data. Technology companies like ours are struggling with a deficit of trust because some of the past generation companies. The way our company works, and I think any company should work, is the customer owns the data. It’s their data. We’re a steward of it. We’re trusted to manage it on their behalf.
Now the future of data ownership has complexities because sometimes our customers, which are the manufacturers, ask, “Do I own the data or does my customer or vendor own the data? I’m using an ASM or a Fuji pick-and-place machine: Is it their data or my data? I’m building a product for Cisco; does Cisco own the data, or do I own the data as a contract manufacturer?” I think there is an answer in every case, the originator of the data owns it and data sharing is how this thing works. You’re able to share data. You’re able to make copies of data that are anonymized aggregated. And people can provide value-added services. What’s in the past is people would just soak up data. You don’t even know what they’re doing with it. You don’t even know where it’s gone. That’s not how we do things. That’s not how it should ever be done in manufacturing. What is the future is clear ownership of data and then sharing it with people who provide value to you. “I know why I’m giving you this data because you’re predicting maintenance on my machines. I know I’m giving you this data because you’re helping me predict my capacity and manage my throughput better. I’m so glad that you have a copy of all this data to do that.”
MB: I think that gets to the heart of it. You have to think about it in terms of what the issue is and what the goal is, and if the goal is to be able to refine our processes so that we have the fewest number of deviations possible and we know exactly what’s going on at the machine level and we achieve optimum levels of efficiency, which is always a moving target, then there has to be some level of that. You mentioned trust, but however you come by it, it has to exist in order for this whole process to work correctly. Would you agree?
AS: I would. Data is becoming one of these key means by which we’re transacting value. A customer could work with us and say, “I will give you money, and you can use my data only for my thing but you are not allowed to make a copy of it. You’re not allowed to improve anything Arch does.” Okay, that’s fine. Give us more money for that because you’re only giving us money. Another customer says, “I will give you money and a right to make a copy of this data to improve your algorithms.” Great. You can give us less money and the data.
That’s how it works. It makes perfect sense. People can decide: Do they want to share data or not, but it typically makes sense, because when you share data you pay less money, and that’s being clear about who owns data, being honest about it and valuing it, which wasn’t always done in the past.
MB: This actually happened. I was driving home and I hit a red light at a traffic light and you know it happens every day to everybody. Light turns green. I drove about a hundred yards and lo and behold, another red. Now understand that this was about 10 o’clock at night. Mine was the only car in sight, and all I could think of is, if we can’t get these basic sensors right, what does that say about the need for, or even the future of, AI?
We know that new technologies take a generation to take hold. So can we really expect AI to shrink that pace, or maybe AI will help itself shrink it? Or are we going to go through the natural curves of excitement, anxiety, learning, and rationalization that come with every new potential game-changing technology?
AS: It’s a great example. That is just like downtime on a manufacturing line. The machine went down again; who designed this thing? Well, people designed it and it has faults in it, and they ran out of time to look at all the data and perfectly orchestrate it. Humans in the government or wherever, they ran out of time to perfectly study a better way to synchronize lights because like in your example, you’re like the product going down the SMT line and you’re like, “Why am I stuck? This is so obvious!” But that’s where AI comes in. Obviously I’m an optimist, but we have the data from the product’s perspective. We have the data every single time the product is slowed down. It doesn’t hit the golden cycle time. It hits a block and suddenly we see a traffic jam on the road and we have a new idea, we have a new insight to fix this problem.
Will there be other silly things? Absolutely. In the new language AIs, people call them hallucinations, where you ask it a question and it’s just totally wrong. I’ll give you a funny example of that with manufacturing. ChatGPT has all the data from just the general internet. There is some data related to manufacturing, so you can go to it and say, “I have error code 2213 or whatever it is on my placement machine or my SPI,” and occasionally it knows the answer, because there is actually some public documentation of equipment, and it’ll say, “Oh yeah, that error code means that you’re out of materials and you should do this.” But it has so much other data from random things that sometimes you’ll ask it a question and it’ll just tell you, “Oh yeah, you need to pour water on it.” Just the completely wrong thing. It’ll hallucinate in sometimes totally bizarre directions and it’s totally ridiculous, which again is why these new AIs aren’t a panacea. They don’t solve everything. Manufacturing data, to the quote you gave earlier, it’s big, but smaller in shape compared to all the world’s books. We have to combine general AI with specific data to get the right results for manufacturers.
Being at the front lines of this stuff, I would share a sense of optimism and excitement. I really am extremely excited. Entrepreneurs tend to be optimistic people in general, but I think manufacturing is an industry that has lots of risks, and we have to think about things.
There are a lot of hard things happening in the world right now, like the change of globalization, regionalization. There’s some good reasons for that and there’s also wars and other things, so there’s a lot to be afraid of and think about and try to improve in the world. I really am optimistic about AI. I think it’s one of the bright parts of the world. I think it’s incredibly powerful and it has way more chance to do good than harm, although there’s both and we need to guard it. Compared to the old internet, everything’s out there. You don’t even know what you’ll find. This is like a guidance system. It’s more like the GPS in your car as opposed to a giant stack of maps that you were given for the first time. I really think it has a great potential to do good and be harnessed in manufacturing and beyond.
MB: Electronics design and manufacturing has historically been in a labor crunch. I would argue that AI is needed badly right now to close that gap. Where does Arch fit into this at the user level?
AS: You’re right. The statistics say there’s more than a 100-million-person labor gap worldwide, more than one million in the US, and the National Association of Manufacturing says 53% of those, at least in the US, have one or two years of experience instead of 10. The question is, how can you use technologies like AI to suddenly give guidance and intelligent actions to people who only have a couple years’ experience so they could suddenly level up, be upskilled as if they have five or 10?
I’ll give a practical example of that. We talked before about machines that have error codes and it takes a ton of time and the manuals are kind of boring to read anyway. People tend to get practical experience after five to 10 years. They kind of know, in this plant these are the errors that tend to come up and here’s what I do about them in my particular plant. That means a rework like this one, you can just keep the machine going. Whatever it is, well you can have an AI system that can read all these error codes, can understand when they matter and don’t, and then you can work with the experts in the plant to build these into what we call playbooks. And our system is automatically watching all the machines, all the error conditions, and when they happen it serves it up now maybe to a junior person who’s only just come to the factory with the expert’s guidance attached to it, and so it’s like they’re being copiloted by the combination of the AI and the expert’s written advice. That could allow them to say, “Oh, I can ignore this error code with confidence. Okay, this one, I need to do a recalibration procedure.” They get right to the correct work instead of spinning their wheels. That’s an example of what we call an intelligent action.
MB: In summary, an AI platform like that of Arch’s can help users get up to speed quickly in their native language.
AS: That’s a great point. Both in the native language of, “I’m a beginner. Make this easy for me,” and in their native language of English, Spanish, Mandarin, Hebrew. The new language AIs are extremely powerful at translation. And that’s something that we’ve already been using early on at Arch. Training materials, for example: We have tools where maybe we have a manufacturer who has sites in five or 10 countries just translate those immediately. It used to be that you need to do this in English and the translation tools really weren’t that good. They are now so good that we do local language translations for all our materials and our interfaces. We have our shop floor interfaces translated into different languages, and what we’re about to add are more of our summary analytics insights, also translated into local language.
MB: Because our industry is full of jargon, the translation in my experience was really the most difficult part. We go and present in other countries, and even when you have interpreters you know you’ve hit a head-scratching moment when you get “that look.” And the difficulty when your entire platform is almost faceless is that you don’t get that feedback in real time.
AS: That’s exactly right. I’m so excited about this part because the tools like Arch’s allow us to build these knowledge playbooks. The CEO of Flex, for example, Revathi Advaithi, says, “Excellence anywhere is excellence everywhere.” For a contract manufacturer for example, they think, “I figured something out in this plant,” whether it’s Mexico, India, the US, Germany, and “I need to spread this excellence everywhere.” And at the same time people are all speaking different languages.
The combination of having standardized normalized data and building normalized playbooks with these new language AIs that don’t just translate word for word, they translate thought for thought, and they even put it in the right grammar, the right idioms, for that local language. It’s really upping the ability to simultaneously do local understandability with global playbooks, global standardization.
Mike Buetow is president of the PCEA (pcea.net); mike@pcea.net. PCEA is hosting a free webinar on AI in Electronics featuring experts from several leaders in electronics design and manufacturing software on March 6 at 1 p.m. Eastern.